Dioptra Product Information What is Dioptra? Dioptra is an open source data curation and management platform designed for computer vision, natural language processing (NLP), and large language models (LLMs). It helps users curate valuable unlabeled data, register metadata, diagnose model failure modes, and integrate with labeling and retraining stacks. How to use Dioptra? 1. Curate the most valuable unlabeled data to improve domain coverage and model performance.2. Register your metadata to Dioptra to ensure your data remains with you.3. Diagnose root cause model failure modes and regressions using Dioptra’s data centric toolkit.4. Use active learning miners to sample the most valuable unlabeled data.5. Integrate with your labeling and retraining stack using Dioptra’s APIs. Dioptra’s Core Features 1. Data curation: Curate valuable unlabeled data to maximize model improvement.2. Metadata registration: Register metadata to keep your data secure and accessible.3. Diagnostics: Use a data-centric toolkit to identify model failure modes and regressions.4. Active learning miners: Sample the most valuable unlabeled data with these miners.5. Labeling and retraining integration: Integrate Dioptra with your labeling and retraining stack. Dioptra’s Use Cases #1 1. Improve model accuracy on challenging cases.2. Shorten training cycles by 3x.3. Reduce labeling costs by 70%.4. Curate data at scale systematically for specific use cases. FAQ from Dioptra What is Dioptra? How does Dioptra work? What are the core features of Dioptra? What are some use cases for Dioptra? Is pricing information available? Dioptra Support Email & Customer service contact & Refund contact etc. Here is the Dioptra support email for customer service: [email protected] . Dioptra Company Dioptra Company name: Dioptra, Inc. .
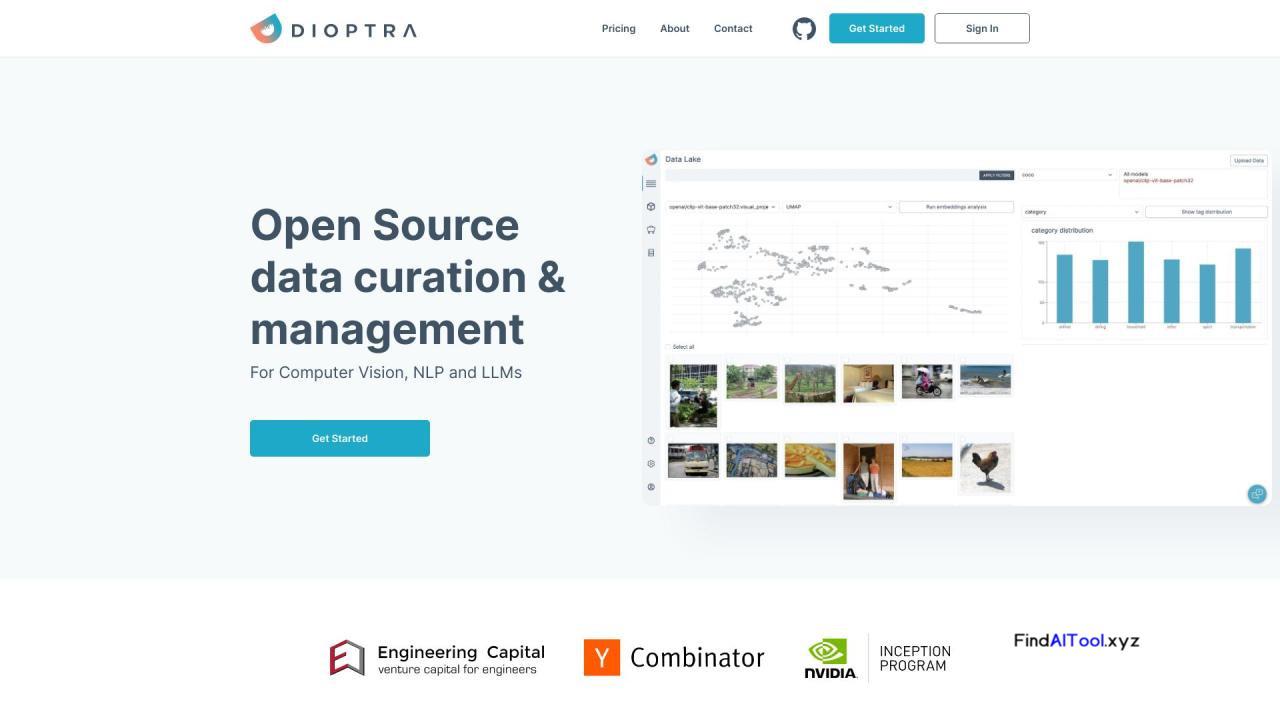
Dioptra
Dioptra is an open source data curation and management platform designed for computer vision, natural language processing (NLP), and large language models (LLMs). It helps users curate valuable unlabeled data, register metadata, diagnose model failure modes, and integrate with labeling and retraining stacks.